Episode #29
Podcast with Steve Miff, Chief Executive Officer, Parkland Center for Clinical Innovation
"Health begins where we live, work, play, pray"
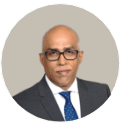
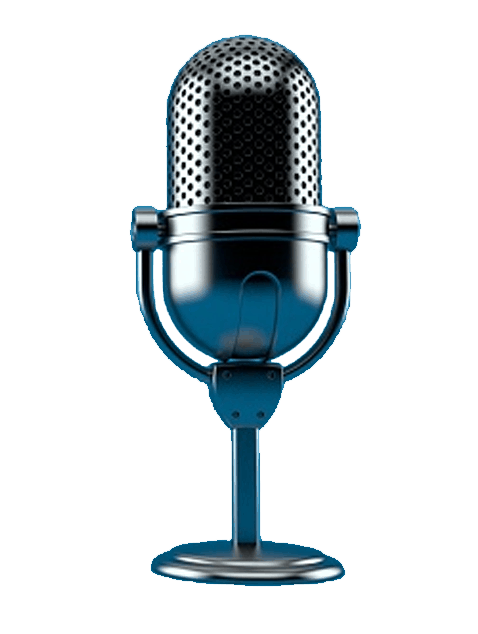
Share
In this episode, Steve Miff discusses how Parkland Center for Clinical Innovation (PCCI), a non-profit organization, has developed advanced machine learning algorithms to understand the role of social determinants of health in vulnerable and under-served communities.
PCCI operates with the assumption that health begins “where we live, work, play, and pray.’ The key aspects of PCCI’s journey have always been to partner with organizations, locally and nationally, who share the same goal and understanding. Steve discusses the success they have had in improving the lives of their communities and reducing the costs of care through their machine learning-based approach. He highlights the importance of a robust data management and infrastructure environment for success with predictive and prescriptive analytics in healthcare.
Welcome to the big unlock podcast where we discuss digital transformation and emerging technologies in healthcare. Here are some of the most innovative thinkers and leaders in healthcare and technology talking about how they are driving change in their organizations.
Paddy: Hello again, everyone. This is Paddy. And welcome back to my podcast. It is my great privilege and honor to introduce my special guest today, Steve Miff, Chief Executive Officer of the Parkland Center for Clinical Innovation. Steve, thank you for joining us and welcome to the show.
Steve: Thank you so much for having me.
Paddy: You’re most welcome. So, Steve tell us how the PCCI came about and your affiliation with Parkland Hospitals.
Steve: Yeah, it’s actually a fantastic story. PCCI started as a department within Parkland back in 2010 with the goal of starting to really look at readmissions and trying to understand the role that social determinants of health and particularly housing instability were playing into the individuals and patients that the parkland was serving. And in 2012, there was the realization that an organization like PCCI would much better operate outside the direct walls of the healthcare system. So PCCI was spun off into an independent, separate non-profit organization that’s still affiliated to Parkland through our board. So, the goal was to not only be able to continue to work with Parkland and the community that Parkland serves, but also enable the organization to reach out and collaborate, partner work with other organizations locally and nationally. So, that was the start of really what I consider to be PCCI. And one other I think really important development in our journey has been that since 2012 we started to build the various models using data science and applying it to social determinants of health and programs and realized that the number of those models had much broad application and commercial value in the marketplace. So, instead of forcing PCCI to be also that commercial entity, we spun off a separate company in 2015 called Pieces Technologies with a completely different company, venture-backed, different structure, leadership, et cetera. But created an exclusive licensing agreement between the IP that PCCI has developed and will continue to develop to license it to a startup organization that can commercialize that expanded, bring it to the broader market in PCCI would continue to be innovation early-stage R&D type of organization, but benefits from those commercial activities. So, I think that’s also kind of adds to our journey, adds to our story. And I think it’s a really important piece of not only our history but how we’re looking to expand and succeed in the future.
Paddy: Yeah. That’s an interesting background. Actually, it’s a very interesting structure that you just described. And of course, PCCI and Parkland Hospital is based in Dallas. And so, I imagine your focus, at least for the near term, is mostly in and around the Dallas area.
Steve: It’s been in Dallas and we’ve continued to expand and work with other entities beyond Parkland in the DFW area. But over the last twelve months, and this is something that’s going to continue moving forward. We’re expanding our partnerships with other organizations across the country and focusing on really staying true to our strengths and mission on applying data science and social determinants of health to those vulnerable individuals and populations, but also be able to partner with organizations to build, test, deploy some of these models in newer and different geographies. I think having that opportunity to really understand how some of the things that are working locally can be adapted in other geographies with similar populations. It’s a key part of our journey and we’ve already started on that.
Paddy: Right. And I’ll come back to that further on in the conversation. PCCI’s focus seems to be on underserved communities. I think you alluded to that in your earlier comments, especially focusing on leveraging social determinants of health to serve underserved communities. Can you maybe give us an example for our listeners to understand how or take any project or initiative where you have used social determinants and your internal capabilities with data sciences and model building and so on to demonstrate positive outcomes for your community?
Steve: No, absolutely. And you are so right. You know, I called sort of the PCCI genius that happens when three things come together. One, that our ability to leverage our data science in AI, machine learning, cognitive computing capabilities apply that to social determinants of health for the high risk, vulnerable populations. And I think a great example of how all those things come together is being focusing on one of the very critical populations that are impacted by socioeconomic status and conditions. And that’s the work in looking at pregnant women who are at risk for preterm birth. And as we’ve really focused on that population, I believe there are three key things that needs to come together for that to be effective. One is to be able to understand the risks so that risk stratification becomes critically important to understand the profile of an individual. So, we can tailor the interventions and engage individuals based on their needs and risk. So, don’t treat each person the same. Number two is to connect individuals to services. What I mean by that is making it easy, make it accessible for individuals to be able to understand not only their risk but understand the options they have available and make those options easily accessible. And number three is also engaged individual directly. We need to and have seen great results in not only connecting providers with the broader community to support those individuals, been engaging individuals themselves, but do that in a customized way based on their needs and risks. So, as we started on this journey, we took the social determinants of health and that became a key predictor in the model. But it was done in a way that we quickly learned that is only powerful when it’s specific enough. And to get it specific enough, you either have to survey, collect information directly from an individual, or use information about the environment, about the neighborhood to understand that local social-economic challenges and then need to be done at the block level, not at the zip code level. So, as we go through that, the journey 12 months results, we risk stratify over 26000 women enrolled over 800 of them into the program. And a key feature of that was a text messaging program to remind them about their doctor’s appointment, provide nutritional tips and other patients’ specific messages. What’s really the main goal was to tailor those and increase prenatal attendance. So, as the old metrics I mentioned, but also pharmacy claims were some of the metrics that went into developing the machine learning-driven predictive model to identify risk-stratified pregnant women. The model included over a hundred and ten features that were contributing to preterm birth, things such as housing, stability, nutrition, nicotine, and alcohol use, whether it’s medical comorbidity, apathetic history, etc. And a one-year pilot we’re actually not only pleased but a bit surprised at how effective it was. We saw over a 24 percent increase in prenatal visit attendance among the women receiving the text messages, and that resulted in over 27 percent reduction in preterm birth at less than 35 weeks of gestation. And that contributed to an over 54 percent decrease in baby costs per member per month. So, you know, sometimes some of these problems are hard to get off the ground and you don’t typically see a ton of results in the first six to 12 months. So, we were particularly pleased to see these fantastic results.
Paddy: So if you were to boil this all down to maybe the one or two big variables that have a very strong correlation towards improved outcomes in terms of everything you described, it is mortalities and increased health for the mother and the baby and so on. What are the one or two that you based on your work of come up with as the most important predictors?
Steve: Yes, absolutely. So certainly, medical history, including the pharmacy utilization and the number three social determinants of health, are the three key elements. But again, the social determinants of health only became part of the top three predictors when that information was specific enough at the block level when it was initially modeled at the zip code level. The social determinants of health factors were outside of the top 10.
Paddy: So social determinants of health are now a big topic. And different people are focusing on different aspects of social determinants. So, as an example, and I’ve heard this being said that a zip code is probably the single biggest determinant of health outcomes for a community. On the other hand, we also hear things about food deserts and transportation deserts. Now we’ve seen Uber and Lyft and all these companies forming partnerships with electronic health record vendors to include the option to actually offer free rides to people who do not have access to transportation as an example. So, when you talk about social determinants, what are the one or two things that for your community that come up with the surface as important ones?
Steve: So, each community varies in our community. What we tend to see is that access to nutritious food tends to be the highest and most prevalent need, followed closely by transportation and housing. However, Paddy, what I will tell you is that we’ve studied this extensively and as we’ve looked at the needs of individuals, not just the primary need, but on average individuals that have one social determinant of health need, they have about 2.5 total needs. So, what I think it’s really important as we talk about these is not only addressing the primary need but concurrently addressing some of those other correlated needs as well. So, that’s one of the key things that I think it’s important to really understand and create an ecosystem and a connected community where these things not only are being understood, but an infrastructure to be able to connect individuals to those services. So, they receive assistance not only for food but equal, like you said, for transportation, for housing, utilities, daycare. And the list goes on and on. But don’t just look at one look at collectively for the comprehensive needs of an individual.
Paddy: So, tell us a little bit about your data sources. Where do you get the data from and what does the data management infrastructure look like?
Steve: Yeah, that’s one of the key things that oftentimes are not talked about a lot because we always jump into the fun part, which is sort of like with our social determinants, all the machine learning. But without the right data and the right infrastructure to be able to manage that data, you can’t really do anything. And I’ll go back to the point that I’ve made earlier, that for us, we have a strong belief and we operate under this principle that health begins where we live, work, play pray. Basically, what we spend our time. It doesn’t begin in our acute care facilities. So, what that translates it from a data perspective is a need to comprehensively understand individuals and digitize that information about not only their healthcare and their health history but information about their life, information about their neighborhood, information about their environment. And I’ll give you some specific examples. But in addition to that digital data, we’re also trying to understand qualitatively the behavioral sciences, also the choices that individuals have to be able to then influence the choices that they make and also better understand the context about their self-capabilities, resources, challenges and some of the things that are starting to be called around learned helplessness, which gets into even one service available, understanding why an individual may not be leveraging and utilizing those. So along with saying that digital information and then qualitative information really important. So, from digital information, I mentioned that we need to understand three key things in their healthcare, including mental behavior health and health history. Information about the neighborhoods and that individual life and bring together those. So, for example, for the healthcare data, the opportunity and the ability to ingest not only claims from whether it’s health plans, state HIS, local HIEs, but equally data from the electronic health records. For that environment be able to ingest and leverage data from local municipalities, whether they’re 311 system 911, as well as data and information from community-based organizations. And increasingly, because so much of the care is transitioning to home, is in just data from IoT devices as well as mobile communication devices. So, a lot of data. Right. And it’s all in different sources. So, then the technology footprint needs to be able to accommodate that. We actually look at licensing that from somebody else. We did that for a short period of time, but quickly realized that there was not really conducive to innovative R&D work that we are doing for actually ended up over the last two and a half years building our own infrastructure in partnership and leveraging the Microsoft Azure cloud environment and their five critical areas that I see and we have seen, they’re really important to be able to manage this. One is secure, encrypted connectivity and point to be able to accommodate the ingestion of all those data sources that I mentioned anywhere from using APIs or fire APIs as SFTPs or databases. In some cases, we just need to ingest spreadsheets because that’s the information that a community-based organization has. You need to have a data engineering and orchestration engine to be able to bring all that information together, align it, fill in the missing data, etc. The fun part is that machine learning-based predictive model environment. What we’ve decided that’s really important is to use as many open source modalities available because that facilitates better knowledge transfer and facilitates much better code creation. But then you need to have a data persistence framework. You also need to have a data dashboarding, reporting framework to be able to accommodate the end-user modalities. And finally, the fifth thing is security and access control. So that kind of spans all of those different things. So complex because of the type of information that needs to come together. But also, I believe it needs to be done in a way that again, facilitates that knowledge transfer and facilitates co-creation because it’s such an emerging space.
Paddy: And that’s, of course, the perfect segue for us to talk about the models themselves and your whole process of developing your own proprietary models or tapping into models that are available as part of your share learning platform. There’s obviously a big opportunity to improve healthcare outcomes through predictive analytics through AI, machine learning, whatever you want to call it. But there is a flip side to this as well and AI has been getting a little bit of attention of late for some of the unintended downsides or consequences, if you will, such as unintended bias. There was one example that was recently quoted in the papers where it was one of the big companies whose models were found to systematically discriminate against certain members of the population. These black-box algorithms are people don’t understand them. And in many cases, it’s not even revealed to them. So, there’s a lot of complex issues around it. Can you help unpack where we are in terms of accepting AI models as important and necessary inputs for improved care delivery? And what should we be cautious about?
Steve: Great. Great question. And you’re so right that there’s been so much attention and AI has become such a buzzword that it feels like everybody says they’re doing it. And as soon as they put it on their portfolio, their valuation goes up tenfold. But I think one of the challenges has been that it’s not being applied or measured than the results have not quite been there. And I believe that there are three key things that need to happen in order for AI to be meaningful given the space that we are on today. One, it needs to be scientifically sound and physician tested. And what I mean by that is the discipline and the rigor around the statistical analysis, the modeling, as well as clinical input into the parameters of the models need to be really sound. And that information needs to be very transparent. That’s not the place to be proprietary. Number two, and you already talked about it. It cannot be a black box. And that black box is not only just the scientific component to it but how it’s being used. You cannot just generate a risk score that tells somebody, hey, this is a high-risk patient without being able to give that individual the reason or the top features that are contributing to that risk. Because by giving those insights from that model, it starts to enable individuals to better understand what’s contributing to that risk and gives them a start where to explore further for better understanding and for action. It actually kind of takes it from just being a predicting model to what I’ll call a prescriptive because you started to prescribe where to actually look next. And the third critical component, which I think has really been missing and we’ve been focusing a lot on, is how do you seamlessly embed any AI or machine learning type model within existing workflows? You cannot expect individuals to use them if they have to go outside of where they’re already doing their work. So, whether that means directly into the electronic medical records or within the workflows of a community based. the organization, the volunteers, etc., But that needs to be a pretty critical component to it. So those three things I think are critical. I think one of the other things, Paddy is that we have been talking about AI and machine learning as this one thing without a lot of attention. When you think about models that not all is the same. And what I mean by that, I tend to think about three different categories. One is more of the supervised time type of methods where we learn to known patterns. I think, you know, anybody that’s doing AI probably knows the majority of models are in that space, you know, takes label input data predicts outcomes. Future things like sepsis models etc., fall in that category. The second bucket is that unsupervised methods ______ [unclear audio] and unknown patterns of much few organizations are doing that. That takes unlabeled input data finds hidden patterns, things such as clustering, or patients like _______ [unclear audio] of analysis falls onto those type of methods. And finally, it’s sort of the whole reinforcement type of models where actually you generate data. So, you take label input data interacts with the environment, learn series of action and starts to actually generate the data. So that’s more of the action derived rewards that are whether it’s chemotherapy, clinical trial, dosing, regiments, election, etc. So, I think folks need to understand that it’s not all the same. You need to really apply the right model to the right area. And also, be thoughtful about what are you applying these in healthcare because there are different applications, whether you’re talking about safety or quality, whether you talk about drug discovery, therapy, diagnostics, administrative, etc.
Paddy: Yeah, you’re probably aware that the FDA is also trying to bring about some they’re proposing some kind of regulation around some of these algorithms so that there is a degree of transparency around what actually goes towards determining the predictors and using them in care pathways and treatment protocols, but also whether there are any changes. There is some kind of a log or trail or some kind of a compliance process in place that helps people understand what change in the input and therefore, what kind of output can work. Quickly do you have any thoughts on that? Are you a part of the process? Are you working with the FDA by any chance?
Steve: No, I am not working with the FDA. We’re actually working closely with CMS and CMMI and I’ve been participating and listening sessions as they’re thinking future models and applications. But no, I have not to date work with the FDA on this.
Paddy: OK, the work that you’re doing is very complex, very advanced. And of course, it requires talent of a certain caliber and data scientists are hard to find and even harder to keep. How do you manage that?
Steve: Yeah, I am always struggling with that because it’s interesting. That’s actually significantly accelerated. It’s been more challenging over the last 18 months. It was before. But the competition for talent in this space and its not just within healthcare, it’s across all sectors. It’s really been difficult and it’s intensified. So, we’re right in the middle of it and we’re focusing honestly on three key things and that’s sort of proven to be somewhat successful. One, we sell our mission and that’s around the opportunity to apply this to help the most vulnerable within a non-profit type of environment while operating within a structure and a similar culture to a technology startup. So, sort of that dichotomy between the application of the work being really close to the impact of the work but having the capabilities of a technology startup very impactful and resonates with individuals. Number two, we actually sell to candidates access to our data and access to and the makeup of our team. So we feel and Dick Daniels, who is the Chief Information Officer for Kaiser Permanente and who is on our board, constantly reminds us that we have unprecedented access to data, not only through the relation that we have with Parkland but with the local Medicaid plan, the local HIE so and the local community. So, we have real-time access and pipelines created to be able to use patient-level data, to be able to build, test, deploy these models. So, we sell that because oftentimes that’s one of the barriers and any geek and data scientist that’s meaningful to them. We sell the team because I think the size of the team is meaningful as well. We have over 10 data scientists led by Vikas Chowdhry, who was the Head of data science for Epic and build that team. We have a very diverse group, so being part of a learning group of peers, it’s something that we focus a lot. And, you know and finally, we have actually started to create our own pipeline and what we’ve done internships in the past. We’ve created a much stronger focus on that. And for example, this past summer, we launch a much more formal internship program that focused on advancing women in data science. And we had seven summer interns. Obviously, all females. So, we took and respond that we did it in partnership with the statistics department at SMU here locally. And it’s been extremely successful. And something that we’re going to be looking to continue moving forward. So, we’re sort of trying to create our own talent pipeline and actually ended up hiring one of those individuals out of this summer program. Something will help is going to continue.
Paddy: Fascinating. Well, Steve, you’ve got some very interesting things going on. And I want to thank you for sharing some of that with me and our listeners. And I really look forward to following your work and PCCI in the coming months and years. All the way best on your mission. And once again, thank you for joining us and being on this podcast.
Steve: Paddy, thank you so much. It’s been a pleasure. And I continue to look forward to learning from your podcast because they are excellent and always very informative.
David: Paddy, thank you very much. Been a pleasure to talk to you today.
We hope you enjoyed this podcast subscribe to our podcast series at www.thebigunlock.com and write to us at info@thebigunlock.com
About our guest
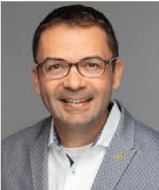
Dr. Steve Miff is the President and CEO of PCCI, a leading, non-profit, artificial intelligence and cognitive computing organization. Before joining PCCI, Dr. Miff served as the General Manager at Sg2, a national advanced analytics and consulting business serving over 1,200 leading healthcare systems. He led the organization’s strategy, operations and growth, and had overall P&L responsibility for the $55M+ business. Dr. Miff also led Sg2’s merger and integration during the VHA, MedAssets and Sg2 acquisition. Prior to Sg2, he was the Senior Vice President of clinical strategy, population health, and performance management at VHA (Vizient Inc.). Dr. Miff was a member of the senior management team and the executive oversight committee. His role with VHA included P&L responsibility across seven national business units with a total of over $25 million in revenue. Dr. Miff launched and grew three new enterprise businesses, led the development and rollout of several key strategic partnerships/acquisitions, and was a senior member of the integration team for the VHA, UHC, and Novation merger. Prior to VHA, Dr. Miff served in various roles at the Rehabilitation Institute of Chicago, National Institute of Standards and Technology, and St. Agnes Hospital System.
Dr. Miff earned his PhD and MS degrees in biomedical engineering and a BA in economics from Northwestern University. He has been an adjunct professor of biomedical engineering for more than five years and has authored more than 100 thought leadership, white papers, and peer-reviewed publications. He has served on the Senior Board of Examiners for the Baldrige National Quality Program and on the Executive Quest for Quality Prize Board Committee for the American Hospital Association. He serves on multiple boards, including DFWHCF, NurseGrid and SMU Big Data Advisory Board. Dr. Miff was The Community Council of Dallas’ 2017 Social Innovator of the Year award recipient and a finalist for the 2019 Dallas Business Journal most admired healthcare CEO award. Under his leadership, PCCI was awarded the 2019 Best Tech Startups in Dallas by the Tech Tribune.
Recent Episodes
About the host
Paddy is the co-author of Healthcare Digital Transformation – How Consumerism, Technology and Pandemic are Accelerating the Future (Taylor & Francis, Aug 2020), along with Edward W. Marx. Paddy is also the author of the best-selling book The Big Unlock – Harnessing Data and Growing Digital Health Businesses in a Value-based Care Era (Archway Publishing, 2017). He is the host of the highly subscribed The Big Unlock podcast on digital transformation in healthcare featuring C-level executives from the healthcare and technology sectors. He is widely published and has a by-lined column in CIO Magazine and other respected industry publications.
Connect